XAI: Explainable AI
Explainable AI: Expanding the frontiers of artificial intelligence
Have you ever asked questions like which is smarter, humans or AI? What might be the next big thing after AI? Is it possibles for humans and computers to get along better, or what in the world is Explainable AI?
Then stay tuned, we're going to dig into the fascinating world of AI. Their strengths and weaknesses, the ways that humans can better work together with them, and, what Explainable AI is.
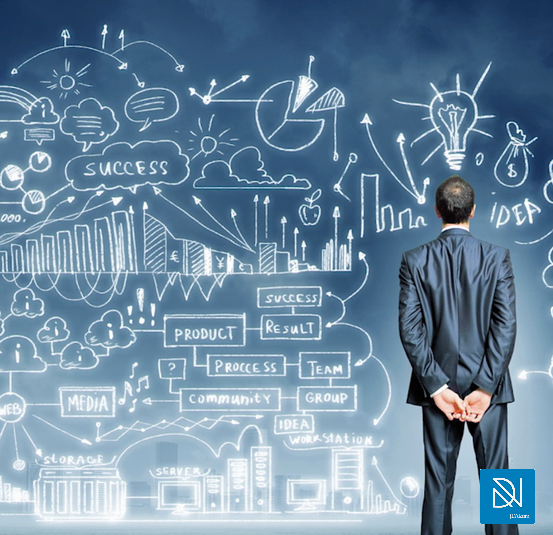
Although AI can be a powerful tool, one of its biggest drawbacks is that it cannot tell you why it made a certain decision or recommendation. It is essentially a black box. XAI is a type of AI that will also give us insight into the reasons why an outcome was recommended. In this article, we'll talk about what it is, how it works, why you might use it, how you could benefit from it, and what you should do about it.
1. Explainable AI(XAI)
1.1 What is XAI
In the normal process of a typical AI system, there are a few steps. First, training data is identified. Then, it undergoes the machine learning process. Then, a learned function or algorithm is produced. Through this algorithm, the system can make decisions or recommendations to a user for a specific situation. However, the user will not know things like, why did the AI do that? Why not something else? When did the AI succeed? When does it fail? When can I trust the AI? How can I correct an error? As can be seen in this diagram, an XAI system could replace the traditional learned function with an explainable model. This model would be built in a way where the decision-making process could be understood by human beings. This process could be presented to the user through something like an explanation interface. As a result, the user could understand why, understand why not, know when the AI succeeds, know when it fails, know when to trust the AI, and know why it made an error. XAI makes the black box, opaque nature of AI a little more transparent. In the following video, we'll cover three of the more common XAI techniques being worked on today.
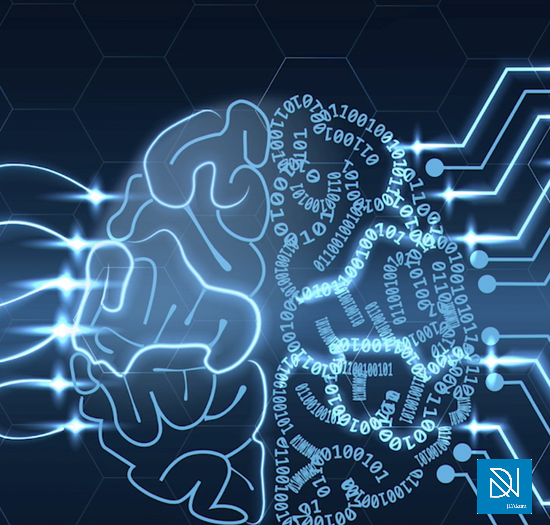
1.2 XAI techniquues
Though the field is early and rapidly changing, three of the more advanced XAI techniques are
- LIME (Local Interpretable Model-Agnostic Explanations) The idea stems from a 2016 paper1 in which the authors perturb the original data points, feed them into the black box model, and then observe the corresponding outputs. The method then weighs those new data points as a function of their proximity to the original point. Ultimately, it fits a surrogate model such as linear regression on the dataset with variations using those sample weights. Each original data point can then be explained with the newly trained explanation model.
- RETAIN(Reversed Time Attention model) RETAIN, was developed at the Georgia Institute of Technology to study models that predicted heart failure. In this method, the model is designed such that, using the data from patient clinical visits, it can predict the occurrence of heart failure at a rate comparable to other models, but also identify which piece of clinical data contributed to the prediction. LRP, or layer-wise relevance propagation, works backwards through a neural network and figures out which input values were the most relevant in coming up with the output. Though these are three common XAI techniques being worked on today, there will no doubt be many others to come as the field develops.
- LRP
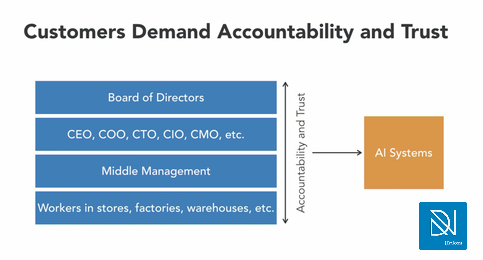
1.3 The needs for XAI - Business
The explainability of AI systems is important for businesses. Two of the core tenants of any successful business are accountability and trust. Customers and partners expect this from frontline workers in the store, factory, or warehouse through middle management all the way to the CEO and the board of directors. As AI systems start making more and more decisions that humans used to make, these tenants will be demanded of these systems as well. AI is now all around us. For consumer applications, it is embedded in things like Waze, Google Maps, Apple Maps, Siri, Alexa, Nest, Uber, Lyft, and so on.
In the enterprise, it is already used in back office systems such as accounting, HR, training and education systems, as well as for marketing, health care, finance, cybersecurity, and a whole host of other applications. For industrial applications, maintenance and operations systems, manufacturing and factory control as well as design systems all incorporate AI.
The penetration of AI into the many facets of our lives will only continue to accelerate over time. As AI becomes more ubiquitous in our lives, there will be more and more areas of risk for AI that will need to be addressed. Examples include trust.
Trust
- Can we trust what the A.I system is recommending to us?
- Can we trust that the systems were not developed using biasd data?
Liability
- What happens when the systems make a mistake?
- Whose fault is it?
Security
- How can we know if systems have not been maliciously manipulated?
Control
- Who(machine or human) has control of a process?
- If machines, then how can human take it back?